We ❤️ Open Source
A community education resource
How AI is making cancer detection faster and more accurate for doctors
Discover Harvard’s CHIEF project and AI’s role in shaping the future of cancer diagnosis.
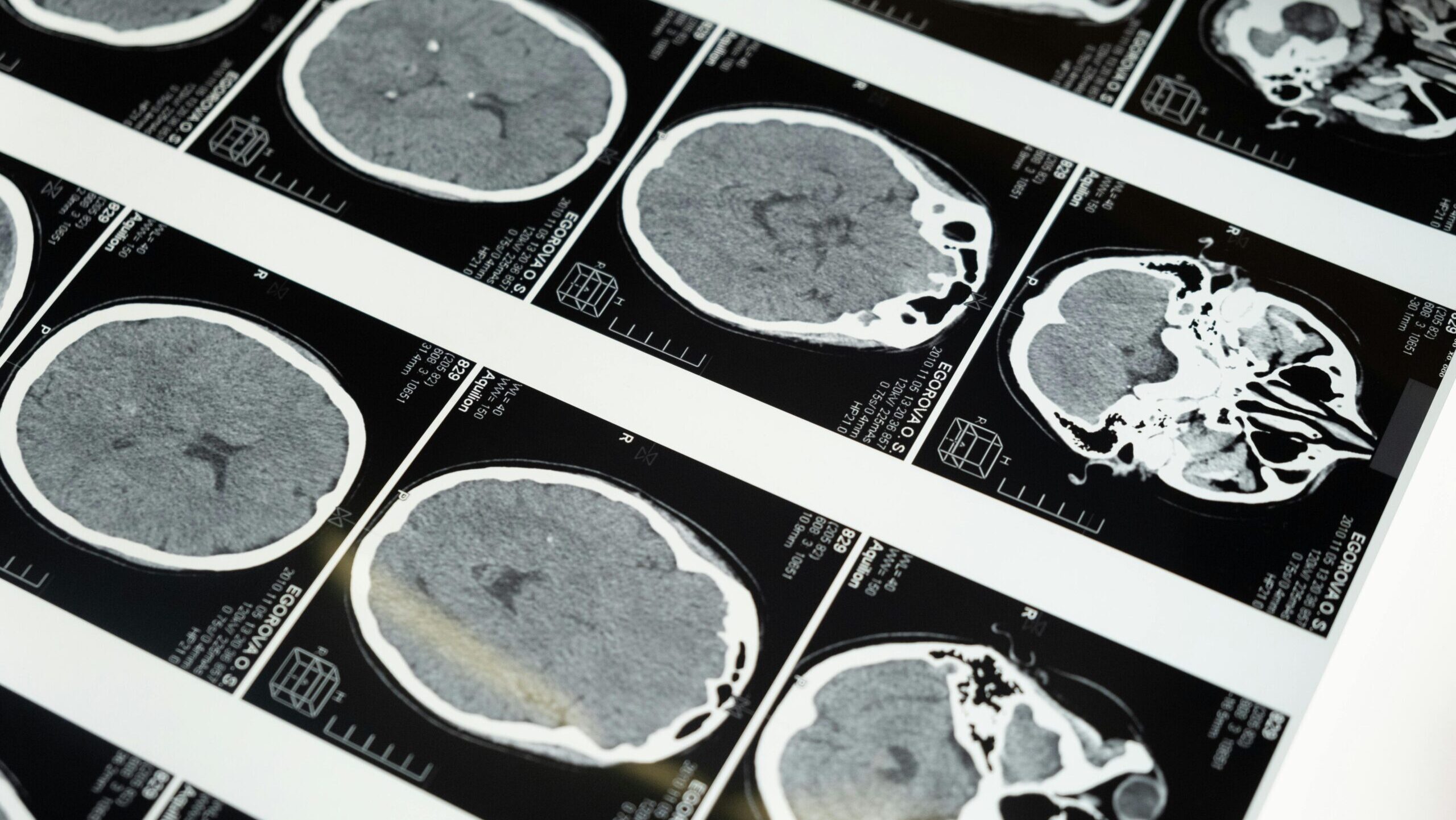
Six years ago, I was diagnosed with cancer. Hearing that diagnosis is truly a heart-stopping moment, filled with anxiety and uncertainty about what lies ahead. Who among us has not lost a relative or friend to this disease? Cancer is pervasive, taking many forms, and the outcomes can often be unpredictable.
Recently, I came across a story that both inspired and fascinated me. A team of scientists at Harvard Medical School has developed an AI-powered tool capable of diagnosing cancer with 96% accuracy. The tool, called CHIEF (Clinical Histopathology Imaging Evaluation Foundation), was trained using 15 million unlabeled images, which were chunked into sections of interest for the model to analyze. It was then further trained on 60,000 whole-slide images of various tissues—lung, breast, prostate, stomach, brain, liver, thyroid, and many others.
What makes this project even more exciting is that it’s open source. It runs on Linux and is licensed under the AGPL. The software is written in Python and leverages Jupyter notebooks for development. Three scientists are behind this ambitious initiative, and the project is publicly available on GitHub, complete with all the prerequisites for running the system.
The Harvard team claims that CHIEF outperforms other state-of-the-art AI methods by up to 36% in key areas such as cancer cell detection, tumor origin identification, patient outcome prediction, and identifying DNA patterns related to treatment response. This achievement isn’t just a technical milestone—it’s a huge leap forward for cancer research and diagnosis. It’s a clear example of the potential of open source AI and the power of collaboration across disciplines.
Intrigued by the implications of this project, I reached out to Dr. Kun-Hsing Yu, one of the lead scientists behind CHIEF, to learn more. Below is the interview I had with him via email.
Why did you choose to develop an open source application? Why did you choose an AGPL 3.0 license?
Dr Yu: We chose to develop CHIEF as an open source application to maximize accessibility and foster collaborative improvement. By making this foundational tool available to researchers and healthcare institutions worldwide, we aimed to enable broader integration and adaptation of CHIEF within diverse healthcare research environments.
The GPL 3.0 license was selected to ensure that any modifications or enhancements remain open sourced, thereby supporting a community of shared innovation and encouraging ethical use.
Why did you choose Ubuntu 18.04 rather than Windows or some other platform? How much RAM and what was the CPU model/architecture for the computer?
Dr. Yu: Ubuntu 18.04 was chosen for its stability and widespread support in machine learning and scientific research. Linux environments, particularly Ubuntu, have extensive compatibility with AI development tools, providing efficient AI model training and easier integration with high-performance computing environments.
We tested CHIEF on many cloud computing platforms and our institute’s compute cluster. The compute nodes in our cluster have 1024 GB RAM shared between 4 GPUs and employ two AMD EPYC 7513 CPUs.
The project has been forked twenty-eight times. Have any of those forks added functionality?
Dr. Yu: Several forks have added functionality. Some have focused on adapting CHIEF to rarer cancer pathology subtypes, while others have applied the framework to datasets involving non-cancerous diseases.
What features are you looking to add to CHIEF?
Dr. Yu: We are currently developing real-time inference capabilities and enhancing interpretability features to help clinicians better understand the model’s decision-making process.
What does this mean for the future of health care?
Dr. Yu: I believe CHIEF represents a major step toward precision medicine by providing a robust and generalizable tool for pathology evaluation. If integrated into clinical workflow, CHIEF can enhance cancer diagnostic accuracy, predict molecular profiles related to treatment responses, and ultimately facilitate personalized and effective cancer treatment strategies
How does CHIEF impact underserved communities?
Dr. Yu: By offering an open source framework that can be adapted to local healthcare contexts, CHIEF has the potential to benefit patients in underserved regions. It could enable healthcare providers with limited resources to access specialist-level diagnostic expertise, thereby enhancing cancer care.
Does this make cancer care more accessible?
Dr. Yu: Yes, it will! Pending regulatory approval, CHIEF will democratize access to cancer pathology diagnostic expertise, making fast and accurate cancer evaluation available to all patients.
What other implications are created by the development of CHIEF?
Dr. Yu: The development of CHIEF has broader implications beyond enhanced diagnostic accuracy. By identifying hidden signals in pathology images, CHIEF uncovered subtle features associated with cancer genomics and treatment responses. Future research could leverage these insights to personalize treatment plans based on the unique pathology of each patient.
What can individuals do to contribute to CHIEF?
Dr. Yu: There are several ways individuals can contribute to the development of advanced AI diagnostic methods. For example, patients can discuss with their doctors the possibility of sharing de-identified pathology images with research institutions, which helps accelerate validation and model refinement. Additionally, individuals can advocate for research initiatives that focus on gathering diverse data. We are currently launching a multi-institutional study that leverages medical data from diverse patient populations, which will enhance the generalizability of AI models.
Be sure to read more about the exciting work of the Yu Lab at Harvard.
More from We Love Open Source
- Getting started with Llamafile tutorial
- How Netflix uses an innovative approach to technical debt
- Evolving DevOps with productivity and improving the developer experience
- Harness the power of large language models part 1: Getting started with Ollama
The opinions expressed on this website are those of each author, not of the author's employer or All Things Open/We Love Open Source.