We ❤️ Open Source
A community education resource
For the good of us all: Charting the future of Open Source AI
Building an inclusive future by embracing collaboration, transparency, and participation.
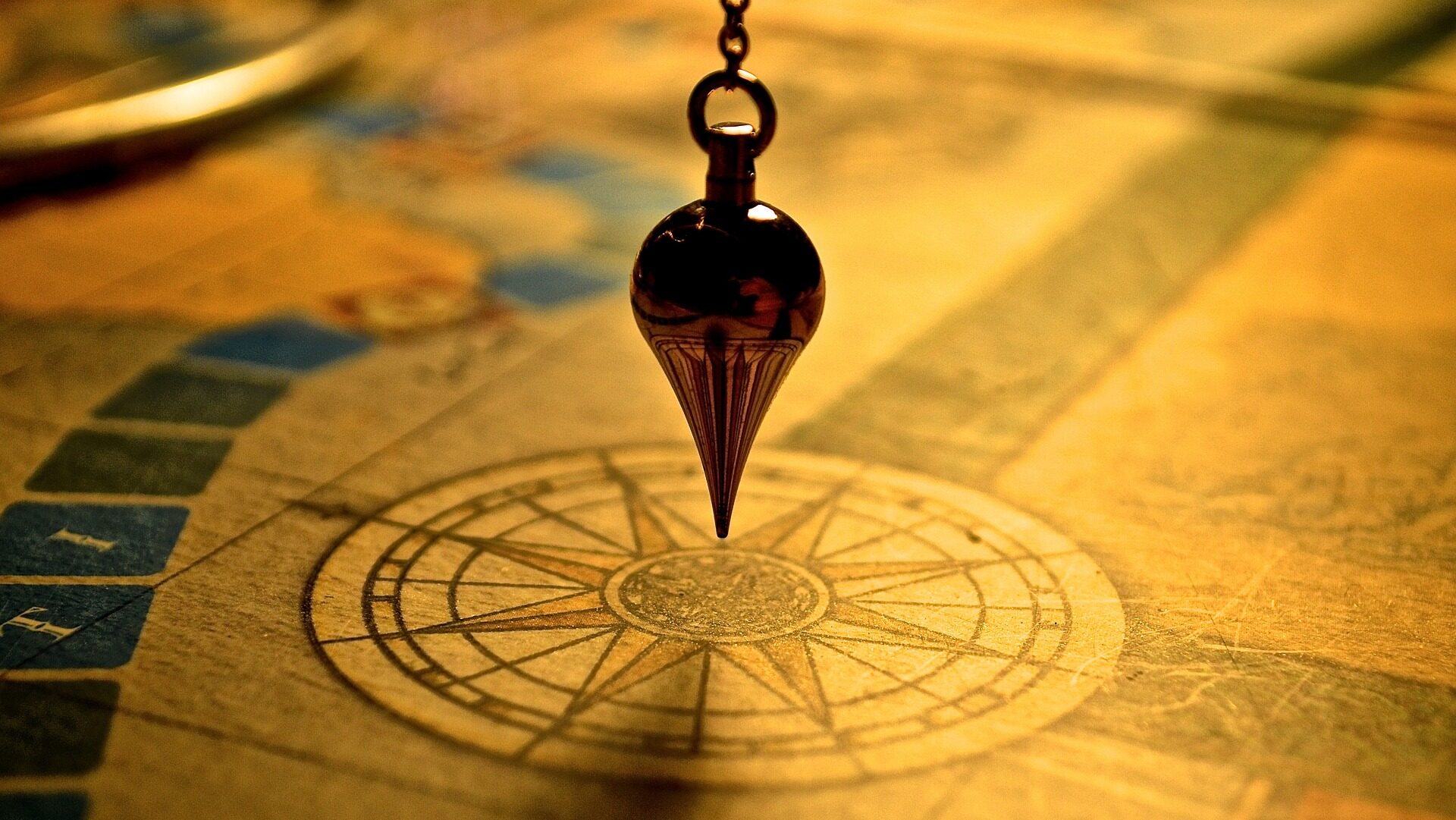
The process of defining Open Source AI exists in an environment of unprecedented complexity but of utmost importance. I want to provide a short background on why the Open Source Initiative (OSI) is doing this, how the work is being done, and why global community—working in a spirit of collaboration—has confidence that issuing the Open Source AI Definition v.1.0 is the correct and most prudent next step in a process to help the open source community make a big leap forward in AI innovation for the good of everyone who relies on the meaning of the term.
The need for a clear definition
Open Source software (OSS) succeeds because anyone can learn, use, share, and improve it without having to ask for permissions. For over 26 years since the term was defined, an entire ecosystem of business, research, and governments around the world has relied on the set of legal documents reviewed and approved for compliance to the Open Source Definition by the Open Source Initiative to build collaboration efforts leading to massive innovation and economic benefits. A 2022 European Commission report estimated that open source contributed between €65 and €95 billion to the European economy (Blind et al. 2021). A 2023 working paper by Harvard Business School (Hoffman et al, 2024) estimates the supply-side value of widely-used OSS is $4.15 billion, and the demand-side value at $8.8 trillion. OSS generates enormous value because it is underpinned by a stable and well-defined set of licenses that are widely understood and adopted.
AI needs similar freedom to flourish.
Unfortunately, as AI and machine learning evolve, traditional open source licenses and definitions fall short in addressing the unique complexities of AI components, especially concerning datasets and machine learning models.
The lack of a clear definition for Open Source AI has led to confusion and misuse of the term. There are many cases, but maybe the most prominent one was Meta’s initial release of LLaMA and LLaMA 2 under terms they labeled as “Open source,” while having restrictive licenses that contradicted open source principles. This ambiguity hinders collaboration and stifles innovation, underscoring the urgent need for a widely accepted Open Source AI Definition.
Our co-design process
The Open Source Definition and the Free Software Definition before were works of lone genius, developed at a time when few people were paying attention to their work. Those Definitions became familiar as more people joined the open source software movement. AI systems, instead, are already under intense scrutiny of legislators, and used by millions of people: the OSI knows that developing a definition of Open Source AI would take a village. Understanding that AI impacts a global community with diverse perspectives, we embarked on the quest to define Open Source AI by adopting a co-design methodology—a participatory approach that shares knowledge and power among all stakeholders (Costanza-Chock, 2020). This method allows us to integrate diverging viewpoints into a cohesive and just standard.
Our journey began in 2022 with extensive research and analysis, including global conversations, podcasts, and panel discussions to map out the key issues in AI under a program the OSI called Deep Dive: AI. In 2023, we hosted in-person workshops across the United States, Europe, and Africa, and facilitated webinars to deepen our understanding. In 2024 we embarked in a global roadshow that took us to 15 cities across continents to gather inputs and feedback. We identified six categories of stakeholders: creators of AI, legal experts, policymakers, integrators, users, and those unknowingly affected by AI.
To ensure inclusivity, we were attentive to diversity, equity, and inclusion. Over 50% of our working group participants are persons of color, 37% are Black, and 28% are women or non-binary individuals. This diverse representation means that the voices creating the definition are globally relevant and equitable.
Confidence in our approach
Despite the challenges inherent in such a comprehensive endeavor, I firmly believe that our co-design process is the optimal path forward. By involving a broad spectrum of stakeholders, we’ve ensured that the Open Source AI Definition reflects a multitude of needs and concerns. Our process isn’t just about drafting a definition; it’s about building consensus and fostering a sense of shared ownership.
Critics have expressed doubts about our approach, suggesting that creating a new definition for Open Source AI could lead to confusion and undermine the essence of open source software and the Open Source Definition that have served us so well for the last two decades. And many open source purists take issue with the current draft of the definition with respect to how it approaches training data. While I respect these concerns, I believe they overlook the unique complexities of AI and the practicalities of data governance.
Addressing data concerns
One of the most contentious aspects in defining Open Source AI revolves around training data. The argument is that without open access to the entire datasets used to train AI models, the principles of open source are compromised. However, this perspective doesn’t fully account for copyright and privacy considerations surrounding data. It also doesn’t take into consideration the practical differences of modern machine learning systems.
Copyright laws vary globally, and the assumption that all training data can be freely shared is not legally sound. For example, the European Union’s text and data mining exceptions allow for AI training but do not permit the redistribution of copyrighted material (White et al., 2024). Mandating the publication of training datasets could inadvertently force developers to violate copyright laws, excluding Open Source AI models from leveraging legal permissions designed to facilitate AI development. It would also permanently put Open Source AI systems at a disadvantage, limiting them to always use smaller datasets and totally excluding the possibility of an Open Source AI built on private data, like medical data.
Instead, the co-design process proposed the concept of “data information.” This would require provision of sufficiently detailed information about the data used to train the system so that a skilled person could build a substantially equivalent system, together with these code requirements:
- A listing of all publicly available training data;
- A listing of all training data obtainable from third parties and where to obtain it, including for a fee; and
- A detailed description of all data, including unshareable data, that provides information about the provenance of the data, its scope and characteristics, how the data was obtained and selected, the labeling procedures and data cleaning methodologies.
The “data information” approach also requires that data shall be made available under terms that allow the copying, modification, and redistribution of the information.
By providing sufficient details about the datasets—such as sources, methodologies, and preprocessing steps—reproducibility is enabled without infringing on legal restrictions. This approach balances the need for transparency with respect for legal boundaries. Coincidentally, it’s the same approach used by Stallman in the GNU GPL:
The source code for a work means the preferred form of the work for making modifications to it. For an executable work, complete source code means all the source code for all modules it contains, plus any associated interface definition files, plus the scripts used to control compilation and installation of the executable. However, as a special exception, the source code distributed need not include anything that is normally distributed (in either source or binary form) with the major components (compiler, kernel, and so on) of the operating system on which the executable runs, unless that component itself accompanies the executable.
Responding to criticisms
It has been suggested by some that without access to all of the exact training data, Open Source AI cannot fulfill the core freedoms of use, study, modify, and share. Concerns have been raised about the potential dilution of open source principles and the risk of confusion with multiple definitions.
On the contrary, the OSI community’s approach applies open source principles to the new realities of AI. The traditional Open Source Definition was crafted with software code in mind, not the intricate interplay of data and machine learning models inherent in AI systems. By tailoring the Open Source AI Definition to address these nuances, we’re creating clarity where there is currently confusion in a complex landscape.
Moreover, insisting on full data transparency without considering legal implications could inadvertently favor large corporations that have the resources to navigate these challenges, leaving smaller players at a disadvantage. Our pragmatic approach seeks to level the playing field, fostering innovation and collaboration across the board.
The way forward
The co-design process we’ve embraced embodies the collaborative spirit of open source, respecting the challenges encountered along the way. By involving diverse voices, carefully weighing thoughtful objections and navigating legal considerations, we’ve developed a stable definition that aligns with open source values while acknowledging the unique aspects of these new AI realities that must be wrestled with.
We recognize that some may view the Open Source AI Definition as less than ideal from a purist standpoint. However, we believe it’s a practical, inclusive, and workable step forward that will enable Open Source AI to flourish and remain relevant. Our goal is to empower a global community of developers, researchers, and users and prevent the monopolization of AI by a few large entities. It is important that we keep in mind our goal — a definition that works for the good of us all — and not let the desire for perfection be the enemy of that very tangible and urgently needed good.
For the good of us all
The Open Source AI Definition is more than a document; it’s a commitment to fostering an environment where AI development is transparent, collaborative, and accessible. By embracing a co-design approach, we’ve crafted a definition that respects legal realities, addresses ethical concerns, and upholds the core freedoms that have driven open source innovation for decades.
The OSI invites and welcomes critical voices to engage with us constructively. That’s what being a part of the open source community is all about. As in any open source community, 100% support of all decisions and outcomes is not expected, but respect for the process is. The challenges we face are complex, but through open dialogue and collaboration, we can navigate them effectively. Together, we can ensure that Open Source AI becomes a force for good, driving innovation and benefiting society as a whole.
More from We Love Open Source
- The Open Source AI definition: Why we need it
- Getting started with Llamafile tutorial
- Harness the power of large language models part 1: Getting started with Ollama
The opinions expressed on this website are those of each author, not of the author's employer or All Things Open/We Love Open Source.