We ❤️ Open Source
A community education resource
The Open Source AI definition: Why we need it
Establishing standards for Open Source AI to uphold open source success and values.
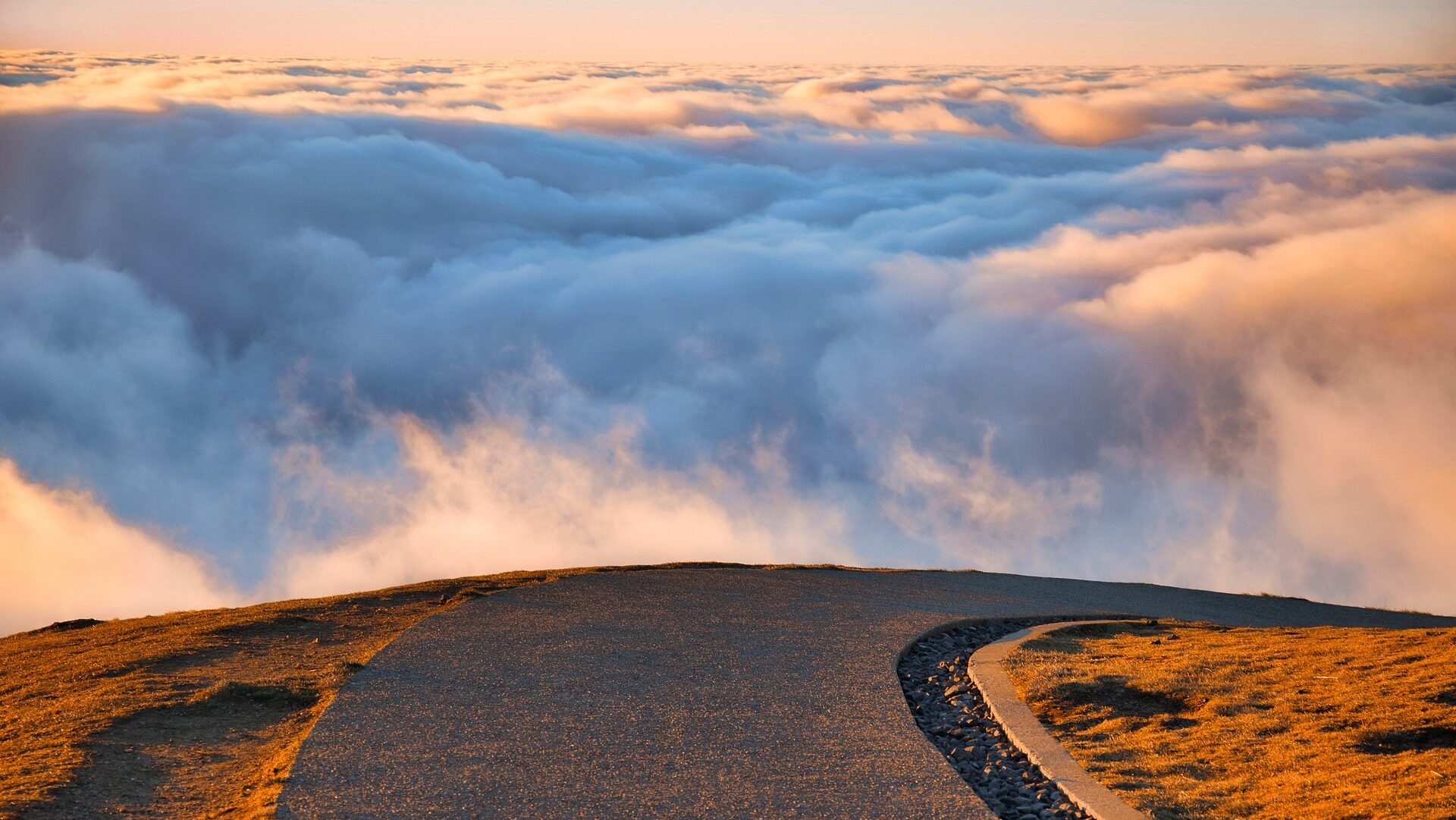
For over 26 years, the Open Source Definition, maintained by the Open Source Initiative (OSI), has fueled permissionless innovation and generated immense economic value. Open source software has opened new markets and allowed global communities to shape the direction of transformative technologies. A Harvard Business School study estimated open source software has a demand-side value of $8.8 trillion, underscoring its vast societal benefits.
I’ve been involved in open source for over a decade, having started the OpenStack project and later the OpenInfra Foundation. With members of our foundation in over 180 countries, I’ve seen firsthand how open source is the most efficient way to drive innovation while delivering economic opportunity to the widest number of people. There’s no question that AI is now redefining computing, with trillions of dollars in investment, and once again we have an opportunity to distribute the future more evenly by embracing open source principles.
This brings me to the Open Source AI Definition (OSAID), an effort organized by OSI over the past two years, which I have participated in alongside others from both traditional open source backgrounds and AI experts. It is often said that naming things is the hardest problem in software engineering, but in this case we started with a name, “Open Source AI,” and set out to define it. As it turns out, that’s just as hard. This difficult work can also appear contentious because people have strong opinions, but that’s nothing new for open source software (or people).
AI systems, however, are more than just software. They involve code, datasets for training, and the resulting weights (parameters) used for inference. Thus, the traditional Open Source Definition doesn’t fully apply to AI, where datasets and weights play a critical role. Despite this, many AI models claim to be “open source AI” simply because their weights are available. But open source is about more than just public access — it’s about permissionless use, something many current AI models fail to offer.
In the absence of a clear Open Source AI Definition, some models marketed as “open source AI” use custom licenses that restrict usage or require explicit permissions, which contradicts the open source ethos. If you have to ask for permission to download or use the model, it’s not open source. The lack of clear standards has allowed some AI developers to mislead the market, a practice largely overlooked by mainstream press covering AI. This is why a clear Open Source AI Definition is needed. Without it, “open source AI” is used ambiguously, often misleadingly, by models that don’t grant the freedoms inherent to open source principles.
As legislation begins to reference “open source AI,” it’s crucial that we have a clear, enforceable definition to prevent misuse and ensure the playing field remains open to all. Additionally, larger players have started lobbying for more regulation, which could benefit them at the expense of smaller, open source options. Without a strong definition, there’s a real risk that this regulation will further entrench the dominance of large companies and stifle the innovation that open source has historically unleashed.
Are datasets like source code?
One of the biggest challenges in creating the Open Source AI Definition is deciding how to treat datasets used during the training phase. At first, requiring all raw datasets to be made public might seem logical, especially for those of us from the open source world. Data, after all, is a key “source” that influences the final AI model, right? That was certainly my first instinct.
However, this analogy between datasets and source code is imperfect and starts to fall apart the closer you look. Training data influences models through patterns, while source code provides explicit instructions. AI models produce learned parameters (weights), whereas software is directly compiled from source code. Reproducing AI models from scratch requires massive resources — upwards of $100 billion for frontier models — while software can be easily recreated with its source code. Additionally, many AI models are trained on proprietary or legally ambiguous data, such as web-scraped content or sensitive datasets like medical records.
Still, data is an essential component of AI systems, and the Open Source AI Definition needs to address it. The most recent drafts of the definition emphasize that any publicly available data used for training should be accessible, alongside full transparency about all datasets used and the procedures followed for cleaning and labeling them. Striking the right balance on this issue is one of the toughest parts of creating the definition, especially with the rapid changes in the market and legal landscape.
Training code & usage restrictions
Two critical requirements for ensuring permissionless innovation in AI, as we did with open source software, are publishing all code used in training and licensing the system under terms without usage restrictions.
While this might seem uncontroversial in the open source software community, today many AI models claiming to be “open source AI” either fail to release their training code or come with bespoke “community licenses” that impose restrictions. Such practices undermine the freedom to use, modify, and distribute the technology. These requirements — full code transparency and freedom from usage restrictions — are likely to be tested soon in the real world, and we need that process to refine and iterate on the definition.
The Open Source AI Definition represents an important step in ensuring that AI models follow the same principles that made open source software so successful. By setting clear standards, we can prevent the misuse of the term “open source AI” and maintain the freedoms that drive permissionless innovation.
What do you think? Read up on the latest spec and join the conversation online.
More from We Love Open Source
- Getting started with Llamafile tutorial
- 4 ways to extend your local AI co-pilot framework
- Building a RAG system with Google’s Gemma, Hugging Face, and MongoDB
- Harness the power of large language models part 1: Getting started with Ollama
The opinions expressed on this website are those of each author, not of the author's employer or All Things Open/We Love Open Source.